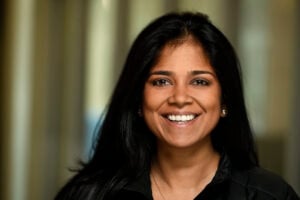
Suchi Saria
John C. Malone Associate Professor
Johns Hopkins University
Research Director, Malone Center for Engineering and Healthcare
Contact: prefix@suffix where prefix=ssaria and suffix=cs.jhu.edu
Twitter: Follow @suchisaria
Other Affiliations: Mathematical Institute for Data Science (MINDS),Institute for Computational Medicine,Laboratory for Computational Sensing and Robotics, Armstrong Institute for Patient Safey and Quality,Center for Population Health Information Technology,and Center for Language and Speech Processing
Formal Bio:See here
Research Interests: At Hopkins, I direct the Machine Learning and Healthcare Lab at Johns Hopkins University. We are interested in enabling new classes of diagnostic and treatment planning tools for healthcare—tools that use artificial intelligence and statistical machine learning techniques to tease out subtle information from “messy” observational datasets, and provide reliable inferences for individualizing care decisions. My work spans the continuum from machine learning foundations and theory to demonstrating novel applications in the real-world to informing policy around safe ML adoption.
Prior to joining Johns Hopkins, I did my PhD at Stanford with the brilliant Dr. Daphne Koller. I also spent a year at Harvard University collaborating with wonderful healthcare informatics researchers Dr. Ken Mandl and Dr. Zak Kohane as a NSF Computing Innovation Fellow. Prior to that, I did research with reinforcement learning pioneers Dr. Sridhar Madhavan and Dr. Andy Barto at UMass. While in the valley, I also spent time as an early employee at Aster Data Systems , a big data startup acquired by Teradata.
At the end of 2018, we spun out Bayesian Health, a health AI startup focused on dramatically improving health outcomes and provider experience. I also sit on advisory boards of several organizations focused on innovative uses of AI or analytics to bring significant societal benefit (see LinkedIn for a partial list).
Example press on our lab’s work:
Recent: Press list
2018 and prior: NSF Science Nation, Baltimore Sun, IEEE Spectrum, Hopkins Magazine, Science, Hopkins Engineering Magazine, Healhcare IT News, Popular Science, NSF Bits and Bytes, Stanford Medicine, Pittsburgh Post-Gazette on the Frontiers meeting, Talking Machines podcast, Popular Science, and TEDxBoston.
Recent: Press list
2018 and prior: NSF Science Nation, Baltimore Sun, IEEE Spectrum, Hopkins Magazine, Science, Hopkins Engineering Magazine, Healhcare IT News, Popular Science, NSF Bits and Bytes, Stanford Medicine, Pittsburgh Post-Gazette on the Frontiers meeting, Talking Machines podcast, Popular Science, and TEDxBoston.
PhD applicants:
If you’re interested in working with me, please apply to the Hopkins program and call me out as a potential advisor explaining why. I get a very high volume of emails from students so I cannot respond to each one individually. If you haven’t heard from me, please don’t assume it to mean lack of interest. Apply here.
Postdoc applicants: Over the last 4 years, the lab has done very exciting work in ML/AI Safety. It has led to 15+ papers (at NeurIPS/ICML/AISTATS/Nature Medicine/NEJM) describing new methods for learning, evaluation, and real-world monitoring. Further, this work has been referenced by several regulatory bodies including the FDA in designing frameworks for AI-based medical devices. I’m accepting postdocs who are interested in advancing the theory, practice and policy around real-world monitoring. If you’re interested, please send me a note.
If you’re interested in working with me, please apply to the Hopkins program and call me out as a potential advisor explaining why. I get a very high volume of emails from students so I cannot respond to each one individually. If you haven’t heard from me, please don’t assume it to mean lack of interest. Apply here.
Postdoc applicants: Over the last 4 years, the lab has done very exciting work in ML/AI Safety. It has led to 15+ papers (at NeurIPS/ICML/AISTATS/Nature Medicine/NEJM) describing new methods for learning, evaluation, and real-world monitoring. Further, this work has been referenced by several regulatory bodies including the FDA in designing frameworks for AI-based medical devices. I’m accepting postdocs who are interested in advancing the theory, practice and policy around real-world monitoring. If you’re interested, please send me a note.
Selected Honors, Awards and Notable Events (only lists awards prior to 2018):
2018
2018
Selected as one of World Economic Forum’s Young Global Leader. To learn more about this recognition, see here.
2017
In National Science Foundation (NSF) Director Dr. France Cordova’s testimony to the Commerce, Justice and Science Appropriations Committee, our lab’s work was one of four pieces of research presented across all areas of NSF (two from CISE) on discussing the NSF budget. It’s a privilege be able to help make the case for increased funding for scientific innovation and research.
2017
2017
Excited to speak on “Machines that Learn to Spot Diseases” at the National Academy of Engineering Frontier’s of Engineering Meeting. More here.
2017
Honored to be included in MIT Technology Review’s 35 Innovators Under 35 (TR35). More here.
2017
Excited to speak on Machine Learning and its Impact at the upcoming National Academy of Sciences Annual Meeting. More here.
2016
Invited Tutorial at NIPS on “ML Methods for Personalization with Application to Medicine.” More here.
2016
Excited to speak on AI and Healthcare at the White House Frontiers Meeting in the National Track. More here.
2016
Excited to speak at the CCC, AAAI and White House’s Office of Science and Technology Policy (OSTP) workshops on the Future of Artificial Intelligence. I gave a talk at the AI for Social Good meeting held in DC on making “meaningful use” of healthcare data using machine learning. More here.
2015
AI’s 10 to Watch. Selected by the IEEE Intelligent Systems once every two years to celebrate “young stars” in the field of artificial intelligence (AI). Selected for research on “Reasoning Engine for Individualizing Healthcare” here.
2016
IJCAI’s Early Career Spotlight. Invited by IJCAI to the “early career spotlight”. Here are the other spotlight presenters.
2015
Science Transtional Medicine Cover article for work on early detection of patients at high risk for septic shock using routinely collected EHR data.
2015
Discovery Award Our work received two (!) of the Hopkins Discovery awards, the first on a new computational framework for large-scale discovery of autoimmune regulators in rheumatic diseases and the second for translating
our models for sepsis. These are highly competitive awards and ours were 2 of the 23 that were selected from a pool of 230 submissions.
our models for sepsis. These are highly competitive awards and ours were 2 of the 23 that were selected from a pool of 230 submissions.
2014
National Science Foundation Smart and Connected Health Research Grant award for developing computational models for prediction in complex, chronic conditions. More here.
2014
Google Research Award for developing machine learning tools for extracting information from electronic health records. More here.
2014
Annual Scientific Award given to the top submission by the Society of Critical Care for our work on early detection of sepsis (selected from 1000+ submissions).
2013
Betty and Gordon Moore Foundation Research award on building safer ICUs. More here.
2011
National Science Foundation Computing Innovation Fellowship; 17 awarded nationally.
2010
American Medical Informatics Association Best Paper Finalist for work on automated annotation of outcomes from electronic health record data.
2007
Uncertainty in Artificial Intelligence Best Student Paper for work on inference for continuous time discrete space models.
2004
Rambus Fellowship awarded for 3 years.
2002
Microsoft Full Scholarship. here.
Selected Publications (partial list prior to 2019; full list in google scholar): ML=Machine Learning, HI=Health Informatics
[ML] A. Subbaswamy, P. Schulam, S. Saria. Learning Predictive Models that Transport. Artificial Intelligence and Statistics (AISTATS), 2019. pdf. NEW
[ML] P. Schulam, S. Saria. Auditing Pointwise Reliability Subsequent to Training. Artificial Intelligence and Statistics (AISTATS), 2019. pdf. NEW
[ML] A. Subbaswamy, S. Saria. Counterfactual Normalization: Proactively Addressing Dataset Shift Using Causal Mechanisms. Uncertainty in Artificial Intelligence (UAI), 2018. pdf. NEW
[ML] P. Schulam, S. Saria. Discretizing Logged Interaction Data Biases Learning for Decision-Making. arXiv preprint arXiv:1810.03025, 2018. pdf. NEW
[ML] P. Schulam, S. Saria. Reliable Decision Support Using Counterfactual Models. Neural Information Processing Systems (NIPS), 2017. pdf.
(Selected for an oral presentation) NEW
(Selected for an oral presentation) NEW
Prior version:
[ML] P. Schulam, S. Saria. Reliable What-If Reasoning with Counterfactual Gaussian Processes. Preprint. pdf. NEW
[ML] H. Soleimani, J. Hensman, S. Saria. Scalable Joint Models for Reliable Uncertainty-Aware Event Prediction. Transactions of Pattern Analysis and Machine Intelligence (in press), 2017. pdfNEW
[ML] H. Soleimani, A. Subbaswamy, S. Saria. Treatment-Response Models for Counterfactual Reasoning with Continuous-time, Continuous-valued Interventions. Uncertainty in Artificial Intelligence (UAI), 2017. pdfNEW
[ML] Y. Xu, Y. Xu, S. Saria. A Bayesian Nonparametic Approach for Estimating Individualized Treatment-Response Curves. pdfNEW
Related work:
[ML] Y. Xu, Y. Xu, S. Saria. A Bayesian Nonparametric Approach for Estimating Individualized Treatment-Response Curves. Conference on Machine Learning and Health Care (MLHC), Aug. 2016. pdf
[ML] Q. Liu, K. Henry, Y. Xu, S. Saria. Using Causal Inference to Estimate What-if Outcomes for Targeting Treatments. NIPS workshop on “What if” Reasoning, 2016. pdf.
[ML] P. Schulam, S. Saria. Integrative Analysis Using Coupled Latent Variable Models for Individualizing Prognoses. Journal of Machine Learning Research 17 (234):1−35. pdfNEW
[ML] D. Robinson*, S. Saria*. Trading-Off Cost of Deployment Versus Accuracy in Learning Predictive Models. International Joint Conference of Artificial Intelligence (IJCAI), 2016. pdf
*equal contribution
*equal contribution
[ML] P. Schulam, S. Saria. A Framework for Individualizing Predictions of Disease Trajectories by Exploiting Multi-resolution Structure. Neural Information Processing Systems (NIPS), 2015. pdf
[ML] K. Dyagilev, S. Saria. Learning (Predictive) Risk Scores in the Presence of Censoring due to Interventions. Machine Learning, March 2016, Volume 102, Issue 3, pp 323-348. Online first: October 2015. pdf, ArXiv
[ML] P. Schulam, F. Wigley, S. Saria. Clustering Longitudinal Clinical Marker Trajectories from Electronic Health Data: Applications to Phenotyping and Endotype Discovery. American Association for Artificial Intelligence, January 2015. pdf
[ML] S. Saria, A. Duchi, D. Koller. Learning Deformable Motifs in Continuous Time Series data. International Joint Conference on Artificial Intelligence (IJCAI), 2011. pdf
[ML] S. Saria, D. Koller, A. Penn. Learning individual and population level traits from clinical temporal data. NIPS Predictive Models in Personalized Medicine, 2010. pdf. (Other versions: short, long)
[ML] S. Saria, U. Nodelman, D. Koller. Reasoning at the Right Time Granularity. Uncertainty in Artificial Intelligence (UAI), July 2007. pdf (Best student paper award)
[ML] V. Jojic, S. Saria, D. Koller. Convex envelopes of complexity controlling penalties: the case against premature envelopment. Artificial Intelligence and Statistics, 2011. pdf
[HI] A. Zhan*, S. Mohan*, C. Tarolli*, R.B. Schneider, J.L. Adams, S. Sharma, M.J. Elson, K.L. Spear, A.M. Glidden, M.A. Little, A. Terzis, E.R. Dorsey, S. Saria. Using Smartphones and Machine Learning to Quantify Parkinson Disease Severity: the Mobile Parkinson Disease Score. JAMA Neurology 2018. Vol. 75, Issue 7, Pages:876-880. pdfNEW
*equal contribution
*equal contribution
[HI] S. Saria. Individualized sepsis treatment using reinforcement learning. Nature Medicine 2018. Vol. 24. pdfNEW
[HI] K. Henry, D. Hager, P. Pronovost, S. Saria. A Targeted Real-time Early Warning Score (TREWScore) for Septic Shock. Science Translational Medicine 2015. Vol. 7, Issue 299. pdf (Cover article)
[HI] S. Saria, A. Goldenberg. Subtyping: What Is It and Its Role in Precision Medicine. IEEE Intelligent Systems, 2015. Vol. 30, Issue 4. pdfNEW
[HI] S. Saria, A. Rajani, J. Gould, D. Koller, A. Penn. Integration of Early Physiological Responses Predicts Later Illness Severity in Preterm Infants. Science Translational Medicine September 2010. Vol. 2, Issue 48. Link (Cover article)
[HI] C. Paxton, A. Niculescu-Mizil, S. Saria. Developing Predictive Algorithms Using Electronic Medical Records: Challenges and Pitfalls. American Medical Informatics Association, 2013. pdf
[HI] S Saria, G McElvain, AK Rajani, AA Penn, DL Koller. Combining Structured and Free-text Data for Automatic Coding of Patient Outcomes. American Medical Informatics Association, 2010. (Best student paper finalist )
[HI] A. J Ma, N. Rawat, A. Reiter, C. Shrock, A. Zhan, A. Stone, A. Rabiee, S. Griffin, D. M. Needham, S. Saria. Measuring Patient Mobility in the ICU Using a Novel Noninvasive Sensor. Critical Care Medicine. Vol. 45, Issue 4. Pp. 630-636. LinkNEW
[Perspective] S. Saria. A $3 Trillion Challenge to Computational Scientists: Transforming Healthcare Delivery, August 2014. IEEE Intelligent Systems. Vol. 29, Issue 4. Link (Invited article)
[Perspective] D.W. Bates, S. Saria, L. Ohno-Machado, A. Shah, G. Escobar. Big data in health care: using analytics to identify and manage high-risk and high-cost patients, July 2014. Health Affairs. Vol. 33, Issue 7. Link ( Short presentation made to an audience of policy makers at the National Press Club, Washington D.C. here .)
National Science Foundation on our work in modeling complex, chronic diseases such as scleroderma. More here.
TEDxBoston talk on Better Medicine Through Machine Learning. More .
NIPS 2016 Tutorial on ML Methods for Personalization with Application to Medicine. More here.
UAI 2017 Tutorial on Machine Learning and Counterfactual Reasoning for “Personalized” Decision-Making in Healthcare. More here. Slides. Video.
Machine Learning and Healthcare Lab:
Peter Schulam (PhD student; Computer Science) NSF and Centennial Fellow
Katie Henry (PhD student; Computer Science) NSF Fellow
Yanbo Xu (PhD student; Computer Science)
Noam Finkelstein (PhD student; Computer Science)
Adarsh Subbaswamy (PhD student; Computer Science)
Andong Zhan (PhD student; Computer Science; Primary advisor: Andreas Terzis)
Hossein Soleimani (Postdoctoral fellow; Computer Science)
Wenpin Hou (Postdoctoral fellow; Computer Science and Genetics)
Anton Alyakin (ugrad; Computer Science)
Tony Wang (ugrad; Biomedical Engineering)
Srihari Mohan (ugrad; Computer Science)
Ryan Newell (ugrad/masters; Computer Science)
Adhiraj Yadav (masters; Computer Science)
San-He Wu (Data Science Staff)
Dhanunjay Singh (masters; Computer Science)
Ethan Groverman (Project Manager)
Yanif Ahmad (Streaming DB Researcher Extraordinaire)
Nestoras Mathioudakis (NIH K-awardee; Assistant Professor in Endocrinology)
Faisal Rahman (Clinical Fellow in Cardiology)
Nishi Rawat (NIH K-awardee; Assistant Professor in Critical Care Medicine)
Alumni:
Andy Ma (postdoctoral fellow 2014-2015; Assistant Professor at Sun Yat-sen University)
Mu-Hsin Wei (postdoctoral fellow 2013-2014; Data Science @ Bloomberg)
Kirill Dyagilev (Postdoctoral Fellow 2014-2016; startup in NY)
Jose Nino (Ugrad 2015-2016; Engineering Infrastructure @ Lyft)
Zhou Ye (2013-2014; Data Science @ Alibaba)
Chris Paxton (2012-2013; PhD student @ Johns Hopkins)
Wenbo Pan (masters student; Computer Science)
Antonia Oprescu (Summer 2014; Undergraduate @ Harvard)
Phillip Oh (Summer 2014; Undergraduate @ Johns Hopkins)
Riashat Islam (Summer 2014; Undergraduate @ UCL)
Ethan Pronovost (St. Paul’s High School; Undergrad @ Caltech)
Other News:
– Student news:
Peter Schulam wins the Centennial Fellowship (August 2013). Miruna Oprescu wins second prize at the JHU Summer Research Expeditions program for her work with my lab on modeling health data (Aug. 2014). Ethan Pronovost selected as one of the finalists at the Americal Medical Informatics Association HSSP for his work with my lab on measuring harms due to false alarms in the ICU (Oct. 2014). Zach Barnes won second prize at the JHU Summer Research Expeditions for his work on deploying a tool for prognosticating lung fibrosis in scleroderma (Aug 2015).
Peter Schulam wins the Centennial Fellowship (August 2013). Miruna Oprescu wins second prize at the JHU Summer Research Expeditions program for her work with my lab on modeling health data (Aug. 2014). Ethan Pronovost selected as one of the finalists at the Americal Medical Informatics Association HSSP for his work with my lab on measuring harms due to false alarms in the ICU (Oct. 2014). Zach Barnes won second prize at the JHU Summer Research Expeditions for his work on deploying a tool for prognosticating lung fibrosis in scleroderma (Aug 2015).
– I’m the workshop co-chair for NIPS 2017 with Ralf Herbrich and NeurIPS (formerly NIPS) with Joaquin Quinonero Candela.
– Old talks (I no longer keep my webpage up to date with talks):
– Invited talks at the Big Data in Biomedicine, Advanced Pharma Analytics conference, Gordon Conference in Health Informatics, and the Technology, Biology and Data Science symposium by Cell Press.
– Interview with Katherine Gorman of Talking Machines
– Invited talks at the iBRIGHT Symposium, University of Oxford, Princeton, Wireless Health, Informatics Meeting at Penn, Emory University, University of Washington
– Area chair for ICML 2016, SPC for KDD 2016
– Check out our workshop on Machine Learning in Healthcare @ NIPS
– Spring-Summer’15 Invited talks at Duke, ENAR, University of Wiscosin, Google DeepMind, University of Pennsylvania, Fred Hutchinson (Data Science to Data Sense Symposium), University of Washington, the VA.
– Daniel Robinson and I won an IDIES seed grant for cost-sensitive predictive tools for preventing in-hospital adverse events (Summer 2015).
– Co-editing a special topics issue for the Journal of Machine Learning Research on “Learning from Electronic Health Data”.
– Senior Program Committee for KDD 2015, IJCAI 2015.
– I lead an invited panel on Predictive Analytics @ American Medical Informatics Association annual symposium (Nov. 2014)
– I was a Keynote speaker on Big data approaches in Health @ the Big Data + Healthcare Analytics Forum by HIMSS (Nov. 2014)
– I presented work on opportunities for big data approaches to improve healthcare at D.C. National Press club (July 2014) at the inaugural event on Big Data by Health Affairs.
– I was invited to the expert’s panel at the Moore Predictive Analytics Symposium (Sept. 2013) to discuss predictive models from EMR and sensing data
– I recently gave an invited talk at the Data Science for Social Good program in Chicago (August 2013)
– I gave an invited panel talk the National Science Foundation and National Institutes of Health joint meeting on Computing and Health; I spoke with three other invited panelists on the ‘Exploiting
Data in Abundance’ panel. (Oct. 2012)
Data in Abundance’ panel. (Oct. 2012)
– I gave an invited presentation at the DARPA Defense Science Office workshop on opportunities in healthcare computing (Nov. 2012)
– I gave an invited talk at INFORMS Healthcare on the big data in healthcare session (July 2013). INFORMS is the largest meeting in Operations Research. Informs Healthcare is a new meeting focused entirely on healthcare applications. There were ~600 attendees to the meeting in its 2nd year.
– I co-chaired ICML workshop on Role of Machine Learning in Transforming Healthcare (July 2013)
– I co-chaired Meaningful Use of Complex Medical Data (MUCMD) Symposium at the Children’s Hospital LA (August 2012)
– Other selected invited talks: Google (Oct. 2013), Carnegie Mellon University (Oct. 2013), Institute for Computational and Experimental Research in Mathematics at Brown University (Nov. 2012), University of Vanderbilt Grand Rounds in Informatics (2012), University of Maryland Machine Learning Seminar (2012), International
Society for Bayesian Analysis (ISBA) (July 2012).
Society for Bayesian Analysis (ISBA) (July 2012).
Teaching:
Current (Spring 15): 600.476/676 Machine Learning: Data to Models
Previous (Fall 13): 600.476/676 Machine Learning in Complex Domains
Previous: 600.476/676 Machine Learning in Complex Domains, 600.775 Seminar in Machine Learning and Data-Intensive Computing
FAQ:
Q00. I am an international student and I want to apply to your PhD program. Are you taking students?
We get 5+ emails of this type per week through the fall. As a once international student, I understand the anxiety of being on the other side. First, these emails are not effective unless you’ve read the faculty’s papers and have something intelligent to say. So, don’t bother wasting your time. Second, let me explain a common issue with PhD admissions for international students. Typically, CS programs tend to fund their PhD students through the length of their program (5 years). This means faculty tend to be risk averse. Having sat on PhD admissions committees, most faculty find it challenging to assess the background of an international student because they don’t often know your school or your advisor. It’s also difficult to gauge whether your grades are highly competitive or not. As a result, most committees pass on international students unless they have an *obviously* strong application. If you’re serious about research and getting a PhD, and don’t have a strong research background (i.e. published papers in top conferences and strong recommendation letters), apply to the masters program. Very often, we take our strong masters students as research assistants after a semester or two. This gives you a chance to build credibility. And, very often, you can recover the cost of your masters through industry internships which pay quite a bit. Also, at a place like Hopkins, there are many faculty outside of computer science that are looking for strong programmers for a research project. That funding can tide you over until you find a lab. But, in the long run, it’s more fruitful to apply to a strong masters program with the goal of switching to a good PhD program rather than going to a PhD program with a poor fit.
Q0. I’m primarily interested in machine learning. But, I’m unsure of the application area. Do I need to have determined this ahead of time?
No. There are a number of faculty including myself that work on machine learning problems applicable to multiple domains. Look through ML@JHU. Also, look through application areas at Human Language Center of Excellence , and IDIES .
Q1. I’m a student at Hopkins and I’m interested in working with you. How can I get involved?
Please take a look at my papers. If you still remain interested, please send me an email. It’s often also helpful to speak with the students in the research group to get a flavor of the problems you could get involved in.
Q2. I’m not at Hopkins currently. Can I apply to your lab for a PhD?
Yes, we are looking for creative and brilliant students to join us. However, you must formally apply to the PhD program for me to be able to consider you. It might be helpful to read through this site on how to put together a strong graduate school application. To gain a better understanding of the types of problems I work on, please read my papers. I soon plan to put up an active projects page but if you’re interested, feel free to send my students or I a note.
Q3. I’m an undergraduate and I am looking for internship opportunities. Can I visit your lab?
Yes, we started a new internship program called the Summer Research Expeditions (SRE) in 2013. The program brings together faculty from multiple departments in engineering and is a great opportunity to gain exposure to multidisciplinary applications of computing.
Q4. I’m looking for postdoctoral or research scientist positions. Are there positions in your lab?
We are always looking for great people to join our group. There is flexibility in terms of the projects you can get involved with. Please send me a copy of your CV if you’d like to learn more.
Q5. I’m interested in machine learning and your work but I have never worked in medicine/biology/healthcare. Do I need a medical background to work on healthcare projects?
No. In my own work, we’ve made significant progress from bringing in a fresh machine learning perspective to existing problems in healthcare. See my recent article to get a flavor of the kinds of interesting computational problems that machine learning researchers can help solve in healthcare. You can learn most of what you need to know about the domain through your readings and interactions with your collaborators. Our healthcare expenses are upwards of 2.5 trillion dollars and we’re in desperate need of better approaches for improving outcomes and lowering cost. Our health system produces vasts amount of messy and heterogeneous data that we need smarter modelers to be looking at and gleaning insights from.
Q6. Why Hopkins?
If you’re interested in solving difficult computational problems in healthcare, Hopkins is one of the best places to join. We have more than two dozen faculty across Computer Science, Statistics, and Biomedical Engineering who are studying novel ways to improve medicine and healthcare using computational techniques. See Institute for Computational Medicine , Lab for Computational Sensing and Robotics , inHealth, ML@JHU and Institute for Data Intensive Science and Engineering for related work by faculty.